Trustworthiness of predictions from statistical and machine learning models
Abstract
Clinical prediction models aim to predict a person’s risk of an outcome (e.g. complications, mortality) given their observed characteristics (1). Risk predictions are traditionally derived from regression models. Artificial Intelligence, and specifically Machine Learning methods, are gaining interest to develop predictive algorithms. Predictions from classic models or AI algorithms need to be reliable to provide valid support for tasks such as shared decision making and whether a person should initiate a particular treatment.
The aim of this talk is to review recent developments in prediction model development and validation. Illustrations will be provided from different medical applications. Trustworthiness will be discussed in relation to the statistical aspects -such as sample size and the exceptionality of patients-, model uncertainty, and heterogeneity between contexts of practical application.
Reference
1. Steyerberg EW. Clinical Prediction Models: A Practical Approach to Development, Validation, and Updating. 2nd ed. Springer International Publishing; 2019. (Statistics for Biology and Health). Available from: https://www.springer.com/gp/book/9783030163983
Vortragender
Ewout W. Steyerberg is Professor of Clinical Biostatistics & Medical Decision Making at the Leiden University Medical Center and Chair at Julius Center for Health Sciences and Primary Care at the University Medical Center Utrecht, The Netherlands.
Termin
Datum: Dienstag, 10. September 2024
Uhrzeit: 11.00 – 12.30 Uhr
Ort: Großer Saal im Hauptgebäude, Deutsches Hygiene-Museum Dresden
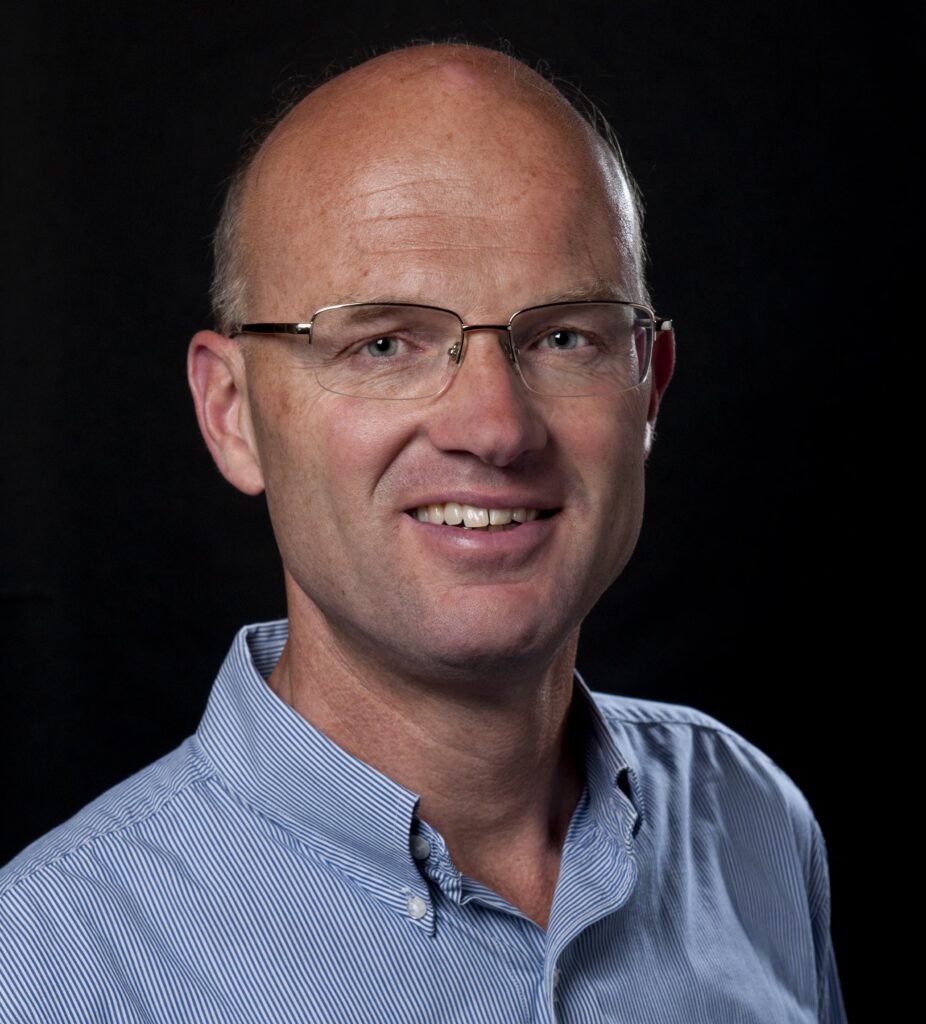